Teaming Up with the Machines: How Can Machine Learning Improve Logistics?
Share:
Welcome to Thomas Insights — every day, we publish the latest news and analysis to keep our readers up to date on what’s happening in industry. Sign up here to get the day’s top stories delivered straight to your inbox.
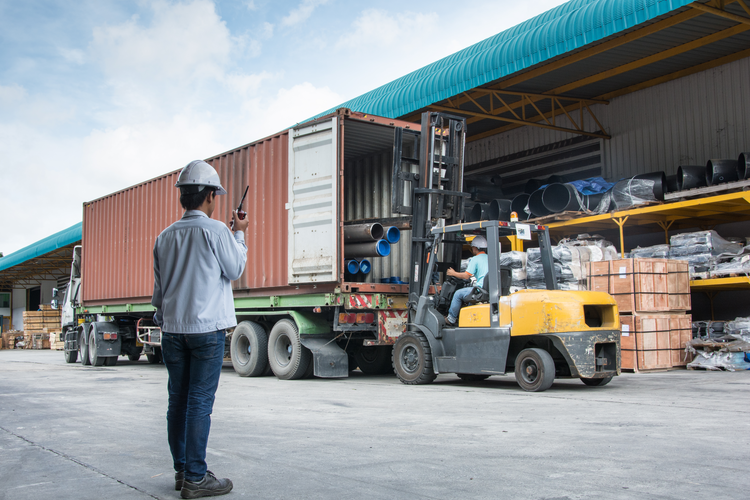
Logistics operations function within an incredibly complex web — suppliers, clients, middlemen, financiers — and depend on everyone providing their supplies, materials, and goods on time. When timing isn’t aligned, the entire web is immediately behind the eight-ball.
As logistics continues on a path of digitization, third-party logistics suppliers (3PLs) are considering the incorporation of machine learning (ML) to help manage the monstrous task of tracking an entire supply chain and keeping processes on schedule.
How Can Machine Learning Change Logistics Operations?
Machine learning can help logistics businesses identify trends in supplier timing and consumer demand. Tasked with picking up orders and delivering them to the endpoint of the supply chain, they often utilize warehouses for storage and midpoint product collection to make the process more seamless.
If carriers can identify trends in supplier delays, they can adjust routing to avoid them and ensure all products are delivered on schedule. Machine learning benefits these companies upstream by making sure suppliers are maintaining their timeline goals based on interactions with other components of the supply chain.
What Can Suppliers Do to Innovate Logistics?
Machine learning requires large amounts of data to function effectively. Suppliers can send this data to 3PLs; the more accurate the data, the better the chance suppliers get accurate timeline estimates. Beyond providing data, suppliers can ensure their shipments are ready to leave when the logistics companies roll in.
Reducing the time carriers burn from waiting for shipments frees up capital. This can be used to develop and implement new systems. For example, a few years ago, some companies began testing platooning, a computer-guided close proximity drafting strategy.
What Types of Data Do Logistics Companies Need for Machine Learning Implementation?
To effectively utilize machine learning, 3PLs need vast amounts of information, including:
- Order size and frequency: How much cargo will be loaded, and how often are these shipments expected?
- Load weight: How much does each shipment weigh?
- Load preparation time: How long will it take to prepare the shipment?
- Shipment departure timing: How long is the logistics company waiting at the pickup site before they hit the road?
ERP systems can generally be used to supply the necessary information to machine learning models with accurate historical information. Past trends help machine learning models make predictions about future demand.
Can Logistics Companies Use Machine Learning Without Supplier Support?
Machine learning systems are incredibly data-hungry. They consume information at an increasing rate as they refine their inner workings. Accuracy and speed increases allow them to generate increasingly higher ROI as they keep learning.
3PLs can use machine learning without data contributions from suppliers, but they may suffer in terms of demand prediction, lose time waiting for cargo, and experience other operational limitations. The training process will also take longer if suppliers don't contribute.
Image Credit: Golf_chalermchai / Shutterstock